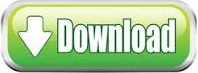
However, their evolution depends on many factors at different scales, altitudes, and times, which leads to modeling difficulties ( Emanuel, 2003). The ability to forecast their trajectory and intensity forecasts is crucial for the protection of people and property.
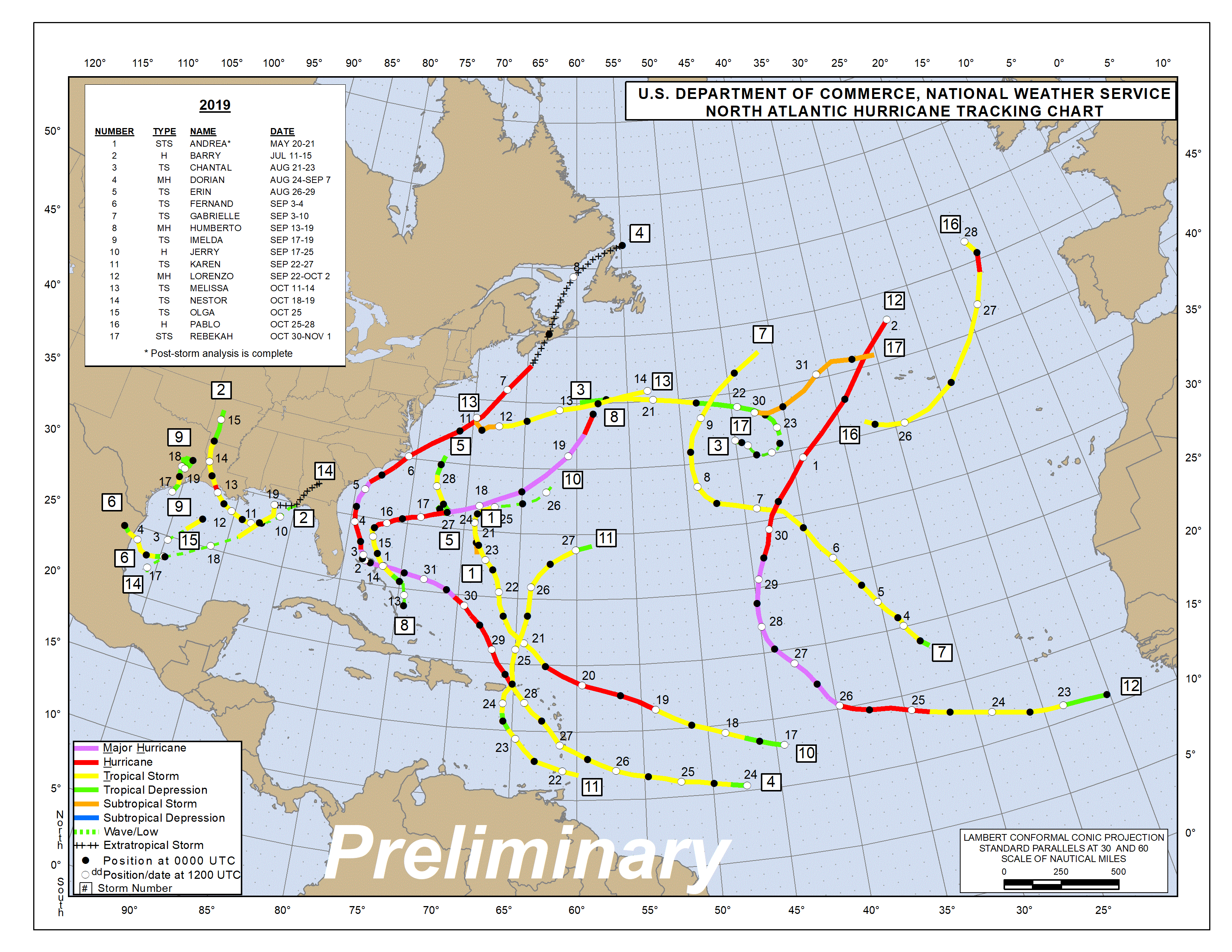
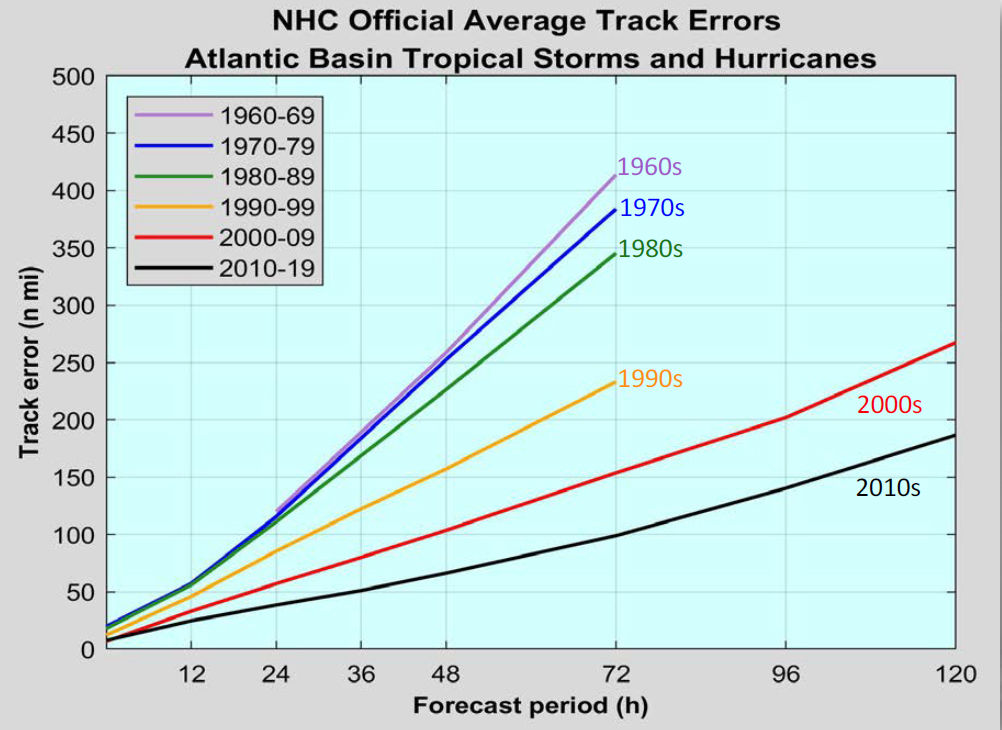
Moreover, our method can give a forecast for a new storm in a few seconds, which is an important asset for real-time forecasts compared to traditional forecasts.Ĭyclones, hurricanes, and typhoons are words designating the same phenomena: a rare and complex event characterized by strong winds surrounding a low pressure area. The advantage of the fused network is demonstrated and a comparison with current forecast models shows that deep learning methods could provide a valuable and complementary prediction. The network is trained to estimate the longitude and latitude displacement of tropical cyclones and depressions from a large database from both hemispheres (more than 3,000 storms since 1979, sampled at a 6 h frequency). We use a moving frame of reference that follows the storm center for the 24 h tracking forecast.
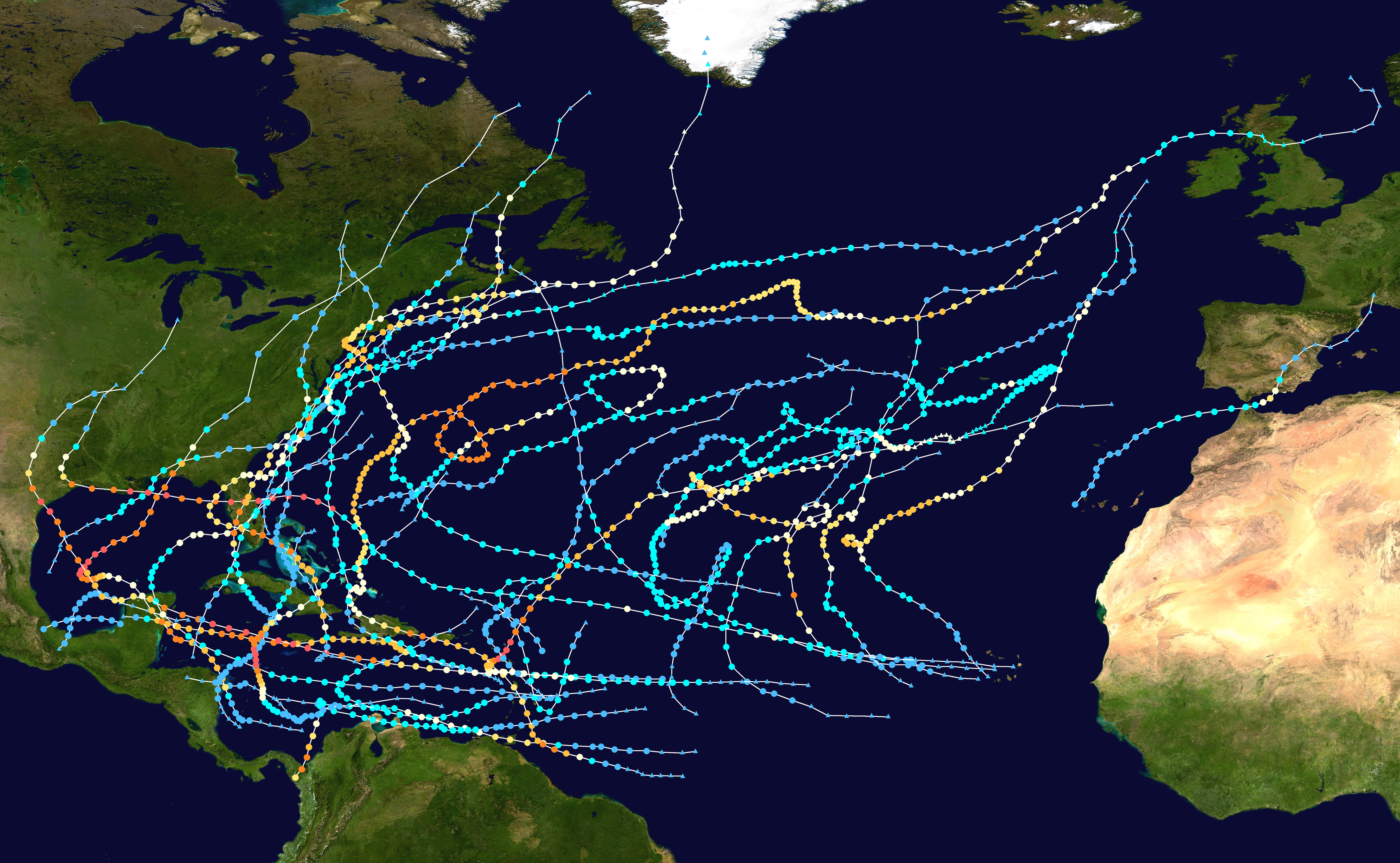
We propose a neural network model fusing past trajectory data and reanalysis atmospheric images (wind and pressure 3D fields). Machine learning methods, that can capture non-linearities and complex relations, have only been scarcely tested for this application. Although forecast dynamical models can provide high-precision short-term forecasts, they are computationally demanding, and current statistical forecasting models have much room for improvement given that the database of past hurricanes is constantly growing. The forecast of tropical cyclone trajectories is crucial for the protection of people and property. 5NOAA/OAR/ESRL/Global Systems Division, Cooperative Institute for Research in Environmental Sciences, University of Colorado Boulder, Boulder, CO, United States.4Inria Saclay–Ile-de-France, LRI, Université Paris-Sud, Palaiseau, France.3Linear Accelerator Laboratory, Université Paris-Sud, CNRS, Île-de-France, France.Savoie Mont Blanc, CNRS, IRD, IFSTTAR, ISTerre, Grenoble, France 1Computer Science Department, University of Colorado Boulder, Boulder, CO, United States.

HAFS is supported under Hurricane Supplemental project - 3A-2: Accelerate Re-engineering of Hurricane Analysis and Forecasting System (HAFS).Sophie Giffard-Roisin 1,2 *, Mo Yang 3, Guillaume Charpiat 4, Christina Kumler Bonfanti 5, Balázs Kégl 3 and Claire Monteleoni 1 It will provide an advanced analysis and forecast system for cutting-edge research on modeling, physics, data assimilation, and coupling to earth system components for high-resolution TC predictions within the outlined Next Generation Global Prediction System (NGGPS)/Strategic Implementation Plan (SIP) objectives of the Unified Forecast System (UFS). HAFS will provide an operational analysis and forecast system out to 7 days for hurricane forecasters with reliable, robust and skillful guidance on TC track and intensity (including RI), storm size, genesis, storm surge, rainfall and tornadoes associated with TCs. HAFS will be a multi-scale model and data assimilation package capable of providing analyses and forecasts of the inner core structure of the TC out to 7 days, which is key to improving size and intensity predictions, as well as the large-scale environment that is known to influence the TC's motion. One of the key strategies defined in the revised Hurricane Forecast Improvement Plan (HFIP) in response to the proposed framework for addressing Section 104 of the Weather Research Forecasting Innovation Act of 2017 is to advance an operational Hurricane Analysis and Forecast System (HAFS) at NOAA/NWS.
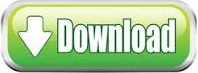